How to Choose the Right Metrics to Evaluate Experiments
Choosing the right metrics is hard. Here's how to structure metrics evaluation frameworks for your experiments that helps you make trustworthy decisions.
In 2012, a Bing employee had a simple idea: make ad headlines longer by combining them with the first line of text below.
Nobody thought much of it.
The feature languished in the backlog for six months until a developer decided to test it. Within hours of launching the A/B test, alerts started firing - revenue was too high. Something had to be wrong.
But nothing was wrong. That simple change ended up generating over $100M in annual revenue.
It was the best revenue generating idea in Bing’s history. But it was badly delayed and almost didn’t launch.
The takeaway? It's extremely hard to predict which product changes will succeed. But it's dangerously easy to think we can.
Introducing Ronny Kohavi
I'm thrilled to have Ronny Kohavi as the editor on this piece. As former Technical Fellow and Corporate Vice President of Analysis and Experimentation at Microsoft, Vice President and Technical Fellow for Relevance and Experimentation at Airbnb, and Director of Data Mining and Personalization at Amazon, Ronny has literally written the book on trustworthy online experiments.
Ronny teaches a top-rated two-week cohort-based course on A/B testing, where you’ll master designing & analyzing A/B tests to grow your business. I just finished it myself - and used the information to write today’s post.
Check out Accelerating Innovation with AB Testing and use my link to get $100 off.
Most Experiments Fail
At companies like Microsoft, Google, and Amazon, thousands of A/B tests run simultaneously. The vast majority fail to statistically significantly improve the metrics they were designed to improve:
Of the experiments run at Microsoft, only about 1/3 improve the metrics they were designed to impact.
At Booking.com, Google Ads, Netflix, and Bing, that number drops to 10-15%.
When Ronny was at Airbnb, 8% of the experiments the relevance team created statistically significantly improved the key metrics.
Yet without rigorous experimentation, we'd ship the wrong features and mis-prioritize. We'd optimize for executive opinion over user behavior. We'd build based on intuition rather than evidence.
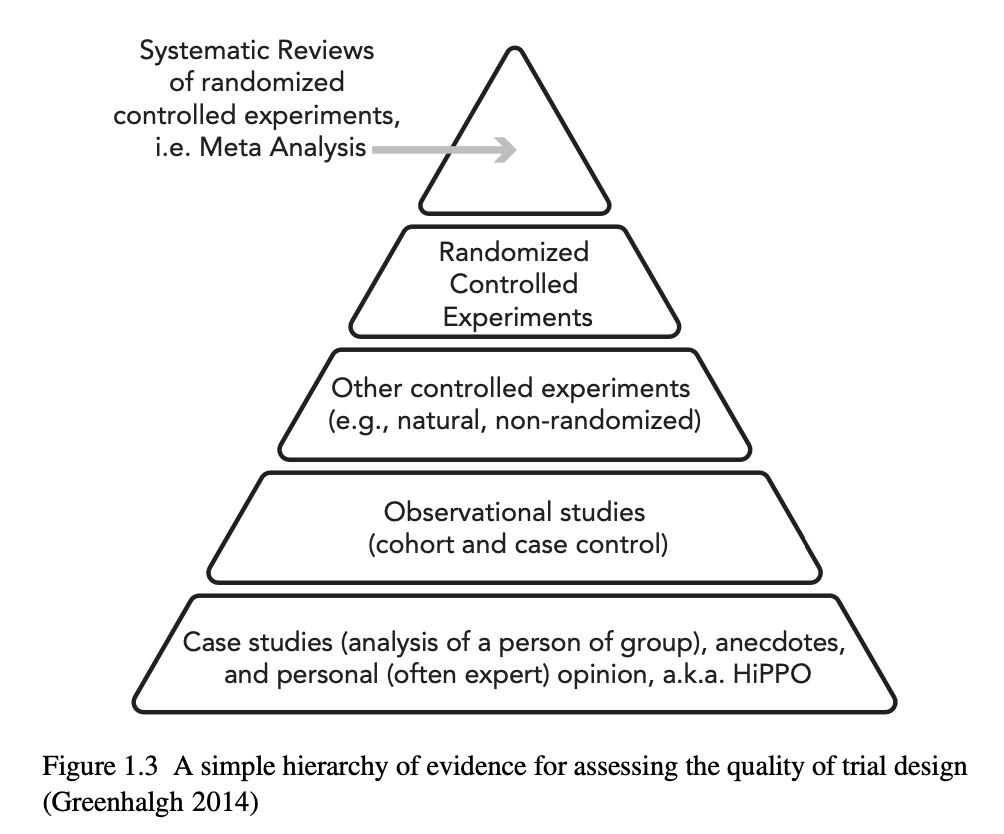
Surprising results provide knowledge that helps generate new ideas and reprioritize the roadmap/backlog. Bing didn’t think small UI changes could make a big difference to revenue until the UI experiment with long ad titles above.
Experiments are the key to gaining new KNOWLEDGE.
This is why measuring experiments correctly is perhaps the most critical capability a product organization can develop. But doing so isn't easy.
Today’s Post
We’re going to break down everything you need to know about measuring experiments correctly:
What Makes a Trustworthy Metric
How to Structure a Good Metrics Framework
Real Examples of Metrics Frameworks
Advanced Topics in Selection
Most Common Mistakes
1. What Makes a Trustworthy Metric
Before diving into which metrics to choose, we need to understand what makes a metric trustworthy.
The right metrics not only measure what you think they measure, but also resist common data traps that can lead you astray.
Getting numbers is easy; getting numbers you can trust is hard.
Whenever you choose potential metrics, think STEDII:
S - Sensitivity
Can your metric detect small but meaningful changes?
Most product improvements deliver just 2-5% lifts, not 50% jumps. High-variance metrics like revenue often miss these small wins completely.
Here’s how to think about good vs bad metrics:
Keep reading with a 7-day free trial
Subscribe to Product Growth to keep reading this post and get 7 days of free access to the full post archives.