Your Guide to AI Product Strategy
Many teams are getting AI product strategy wrong. You see it everyday. Here's, step-by-step, how to build a really effective one.
Everyone needs an AI product strategy.
It doesn’t matter if you’re an AI company, a new-school software company, or an old-school company undergoing transformation.
Investors are demanding an AI strategy, and companies are delivering.
Introducing Miqdad Jaffer
I’ve decided to partner with a true leader in AI - Miqdad Jaffer. Miqdad was a Director of AI at Shopify and now is a Product Leader at OpenAI.
Miqdad also teaches a 6-week cohort course, the AI PM Certification. Get $500 off his course using my code AAKASH25. The next cohort starts April 26th.
The Hard Truth
Let's get real.
Most AI product strategies we have are doomed from the start.
They fall into one of four major traps:
"Sprinkle some AI on it" approaches that add minimal value but maximum complexity
Technology-first fantasies with no clear user problem
Copy-paste jobs that mimic competitors without strategic differentiation
Hallucination factories that prioritize cool demos over solving actual problems
Why?
Because product leaders and companies are force-fitting their old strategy playbooks to AI.
AI isn't just a new tool in your toolbox—it completely reshapes what's possible and how users interact with your product.
The result is that building an AI product strategy is an art itself.
I’ve already covered how to build a great product strategy.
Today’s Post
We’re going to give you all the tools you need to create a stellar AI product strategy:
Do You Really Need an AI Strategy?
The History and Future of AI Strategy
Three Non-Negotiable Principles of Winning
🔒 The Strategy Framework That Actually Works
🔒 Common Strategy Pitfalls and How to Avoid Them
🔒 Practical Examples of AI Strategy at Each Step in The Stack
1. Do You Really Need an AI Product Strategy?
Before we go further, let's be honest about WHY most AI product strategies stink:
Product teams start with "How can we add AI?" instead of "What user problem are we actually solving?"
The result is feature graveyards filled with abandoned AI experiments that users never asked for and didn't need.
So, first ask yourself: where do I really need AI strategy?
We’ve built the AI Product Decision Framework to quickly help:
Now that you know where you need an AI Product Strategy, let’s get into the meat of it.
2. The History and Future of AI Product Strategy
Understanding AI strateegy’s past, present, and future isn’t just academic—it’s the foundation for crafting a strategy that turns AI into a competitive edge.
Give us a second.
The Past: ANI
Product leaders have actually been building successful AI strategies and products for the past 20 years!
Web search algorithms
Personalized recommendations on streaming platforms
And smart speakers
They all represent narrow AI applications that have become increasingly sophisticated within their specialized domains.
These are specialized tools, brilliant within their lanes but useless outside them. A chess AI can’t drive a car, and a speech system won’t curate your playlist.
Strategically, narrow AI taught us that: focusing on a well-defined user problem—not flashy tech—drives success.
The business impact proves it:
Amazon attributes 35% of its revenue to its recommendation engine.
Netflix saves an estimated $1 billion annually through its recommendation algorithms by reducing churn.
Google's search algorithms process over 8.5 billion searches daily, each one a specialized AI task.
So isn’t it funny so many people are forgetting this lesson?
The Present: Generative AI
Why we’re writing this piece now is because of the recent advances, of course, in generative AI.
Forward-thinking product teams aren’t slapping generative AI on as a gimmick—they’re reimagining entire strategies around it.
Take these domains:
Content creation - Easygen accelerates LinkedIn posts
Code generation - GitHub Copilot boosts developer productivity
Design prototyping - Runway streamlines creative workflows
Generative AI shifts the strategy playbook from personalization to augmentation.
Your product strategy must identify where creation bottlenecks exist in your user journey and use generative capabilities to unlock value.
The Future: AGI
The future is AGI—systems rivaling human intelligence across domains.
We’re not there yet, but strategists need to anticipate the road ahead. Here’s the progression we expect:
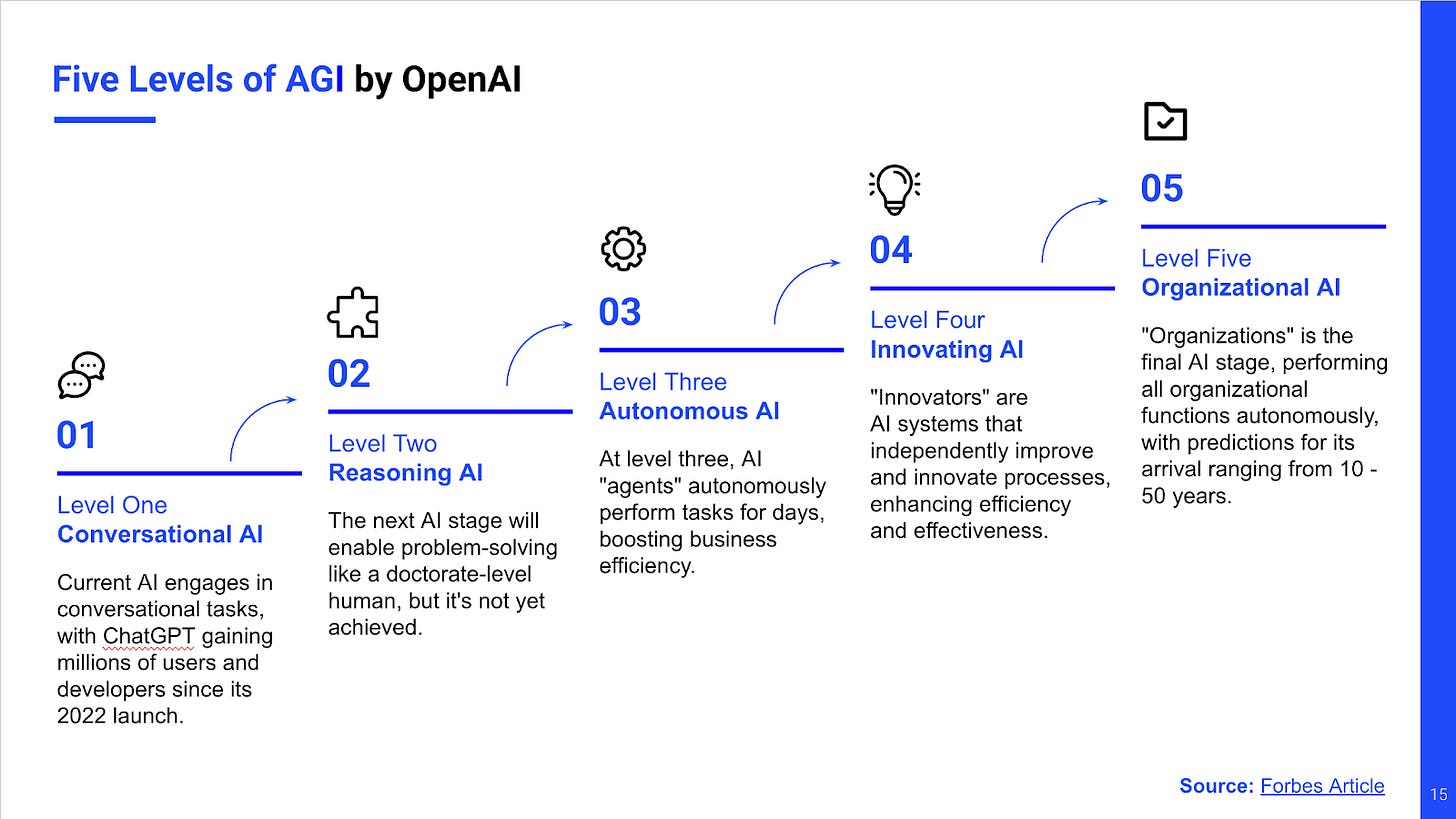
Level 1 AI can engage in natural-sounding dialogue and perform simple tasks. However, these systems frequently make factual errors, struggle with complex reasoning, and lack the ability to plan multi-step actions effectively. Think GPT-2.
Level 2 represents our current position—AI systems that can solve problems with the sophistication of a doctorate-level human. These systems truly understand and reason through complex problems.
For product strategy, this is the sweet spot: they’re ready for high-value use cases—think advanced analytics, decision support, or personalized experiences—provided you’ve got robust data and oversight to keep them on track.
At Level 3, agents will be capable of independently performing tasks over extended periods. We expect them to:
Research, plan, and execute an entire marketing campaign
Develop a software application from specification to deployment
Conduct scientific research, generate hypotheses, and design experiments to test them
But we’re not there yet! (You’ll see more of the implications of that later.)
Level 4 AI systems won't just execute tasks—they'll actively improve and innovate processes on their own.
Level 5 is the final boss stage. At this level, AI systems would become capable of running entire organizations.
The key takeaways for strategists are:
Root in Today: Use Level 2 AI to solve complex, data-rich problems—think analytics, personalization, or decision support.
Prep for Tomorrow: Design for Level 3 agents by collecting proprietary data and testing human-AI handoffs.
Stay Grounded: AGI hype is real, but your strategy wins by delivering value now, not chasing distant dreams.
3. Three Non-Negotiable Principles of Winning AI Product Strategy
So how do you create a great strategy for level 2 AGI, that is ready for level 3?
If you take nothing else from this post, remember these three principles:
Principle 1 - Solve Real Problems, Not AI Problems
❌ Wrong approach: "How can we add AI to our product?"
✅ Right approach: "What user problems can we solve better using AI capabilities?"
Repeat after us: AI is a means, not an end.
The market doesn't care about your clever implementation—it cares about outcomes. Every AI initiative should start with a clear user problem statement and a compelling "why now?" rationale.
You know this because every SaaS app out there has stuffed AI in our faces–and we just ignore it.
That’s why, when Miqdad was at Shopify, the team killed countless AI projects that engineers loved but didn't solve merchant problems.
The AI projects that succeed always have a crystal-clear user impact thesis first, technology second.
Principle 2 - Your Data Is Your Moat, Not Your Model
Most PMs and strategists obsess over model selection.
What they should be obsessing over is data strategy. Foundation models are becoming commodities.
Your unique data and how you leverage it is the true competitive advantage.
Ask yourself:
What proprietary data do we have that others don't?
What unique domain-specific information can we leverage?
How can we create virtuous data loops where product usage improves our AI?
Netflix doesn't win because they have better recommendation algorithms than Amazon—they win because they have better viewing data and engagement signals.
Your AI strategy must articulate how you'll create similar data advantages.
Principle 3 - Design for Human-AI Collaboration
The most successful AI products aren't fully autonomous—they're collaborative.
They make humans more capable rather than trying to replace them.
What this means for your strategy is you must:
Design fallback paths when AI fails
Build confidence through transparency
Create feedback mechanisms that improve the system
Take Cursor–which is one of the fastest growing startups ever, already at $100M ARR.
It succeeds not by replacing developers but by making them more productive.
Your strategy should clearly define how humans and AI work together rather than promising magical automation.
Aakash learned this first-hand when he launched the AI email writer at Apollo: the emails didn’t work. It wasn’t until the team released an AI email collaborator that the feature actually took off.
4. Your Step-by-Step AI Product Strategy Framework
That’s all the high-level theory you need.
Now, here's our battle-tested framework for building an AI product strategy:
Keep reading with a 7-day free trial
Subscribe to Product Growth to keep reading this post and get 7 days of free access to the full post archives.